According to a survey conducted by Qualtrics last year, over 50 percent of decision-makers in market research are convinced they know what AI is in concrete terms. Just about all of them assume that it will have a very big impact on the development of the industry in the coming years.
Moreover, trend researchers at an SAP subsidiary focused on experience management predict that at the latest in five years, at least 25 percent of all surveys will be conducted with the help of digital assistants.
Since the release and spread of ChatGPT and other useful solutions based on large language models (LLM), it has become clear that NLP is rapidly gaining in performance and importance. However, OpenAI’s prodigy has not mastered everything already possible with the help of algorithms.
New NLP tools for documenting and analysing conversations are currently presented to the public almost daily. Properly applied, AI can positively impact not only how we communicate with machines, but also the way we humans work, research and interact with each other. Thanks to rapid innovation over the past years and months, NLP-based software can now also be used to optimise qualitative research effectively.
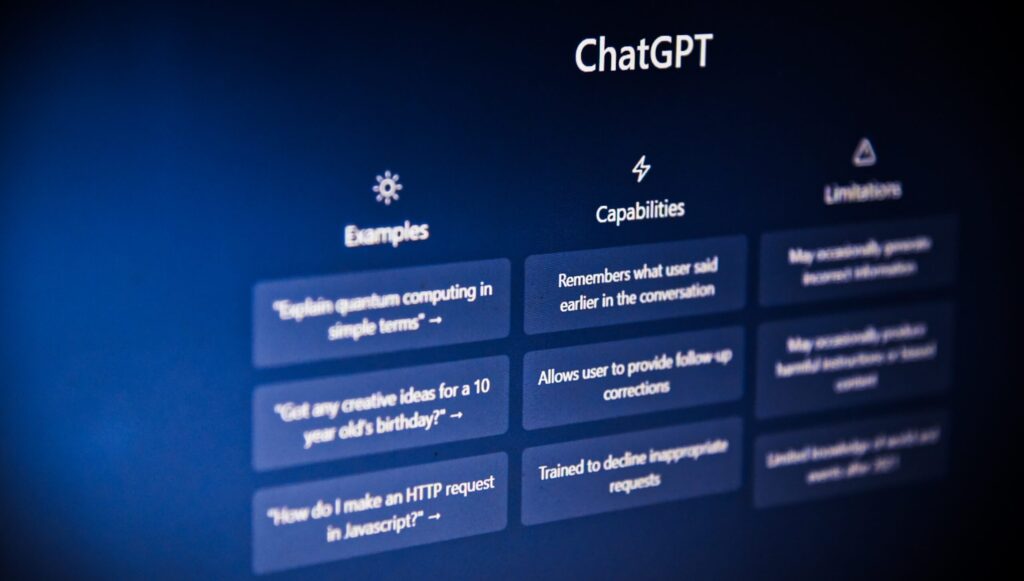
Fundamental methods of NLP
NLP methods and systems for speech recognition and conversational intelligence all have clear strengths and weaknesses, but if they are applied in a targeted, controlled and combined manner, they can deliver a great deal of added value.
The fastest growing area is automatic speech recognition (ASR) which converts spoken words into text. These systems work very precisely and are therefore ideal for tasks such as speaker diarisation, logging and transcription. However, when it comes to recognising multiple speakers in noisy environments as well as accents and dialects, some of these solutions still have their difficulties.
Another innovative NLP element are systems for natural language understanding (NLU), one of the areas in which Tucan.ai is specialising. The technology goes beyond transcribing words and tries to determine the meaning behind them. NLU is perfectly suited for sentiment analysis, encoding, and intention recognition.
Finally, there are conversational AI systems that allow programs to have an informative conversation with a human. Virtual assistants can automatically collect data, generate speech and give personalised answers, but usually still find it difficult to correctly classify subtle forms of speech such as idioms or sarcasm.
You want to test Tucan.ai for your Company?
Benefits of NLP for researchers
Firstly, if developed and applied adequately, NLP can help reduce analysts’ personal biases. Whenever a person perceives something, the content is filtered through subjective experiences and opinions before they can understand it, which naturally introduces a certain kind of bias. A typical countermeasure is to have two analysts working on the same data set, bit this usually takes twice as long and costs much more.
NLP tools are now being increasingly applied by researchers to process, encode and extract findings from interviews and focus groups. One main advantage is the ability to quickly and accurately transcribe large amounts of spoken data and collect free-text data that would else be overlooked.
NLP tools can also be used to analyse transcripts and identify key themes, statements and patterns that are difficult to detect through manual analysis. They help us identify specific nuances of speech, such as tone of voice, intonation and nonverbal cues.
In addition, the automated nature of these AI-powered applications allows us to analyse larger data sets, leading to robuster results in less time. Automating manual processes enables researchers to reallocate certain resources, which makes many of these tools very cost-effective.
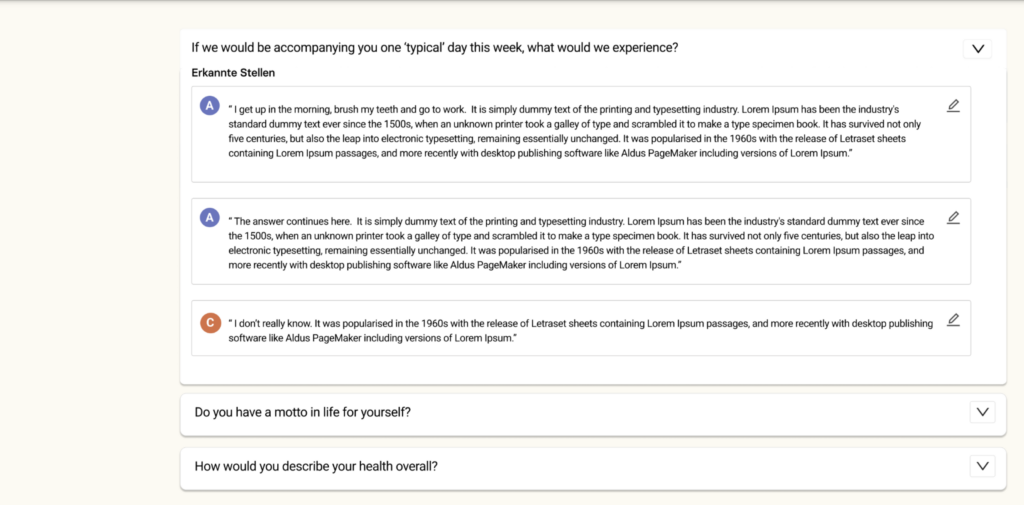
Uses cases in qualitative research
Many leading market research institutes and companies are already making extensive use of NLP. Industry leaders such as GfK, Kantar, Ipsos and GIM apply or offer automatic speech recognition and text analysis tools to transcribe and encode language data to better capture and classify evidence. Continue reading for the most popular and important use cases of NLP in qualitative research.
Data collection and procession
Automated data capture involves reading text and recording, transcribing and summarising conversations. With the help of AI, these tasks can not only be outsourced and accelerated, but also made more accurate, objective and transparent. For example, some tools are able to produce literate (interjections), corrected (grammar) and abstract transcripts as needed, helping to scale interviews and focus groups more easily.
Text analysis
Text analysis and topic recognition are effective and established methods for gaining reliable insights from larger text datasets. IBM estimates, for instance, that about 80 percent of data worldwide is unstructured and consequently unusable. Text analysis uses linguistic and machine learning techniques to structure the information content of text datasets. It builds on similar methods as quantitative text mining, in addition to modelling, however, the goal is to uncover specific patterns and trends.
Topic recognition
Topic modelling is an unsupervised technique that uses AI to tag text clusters and group them with topics. It can be thought of as similar to keyword tagging, which is the extraction and tabulation of relevant words from a text, except that it is applied to topics and associated clusters of information. NLP models can also compare the data they have been provided with – like the results of a study – with similar resources. In this way, inconsistencies can be identified and “gaps filled” as the AI “knows the context” of other data pools.
Sentence embedding
Probably the most effective application case of NLP in qualitative research to date is AI-assisted sentence embedding. It involves the assignment of words to matching or meaningful opinions, essentially facilitating the processing of large amounts of data and automated extraction of the most important statements from qualitative interviews.
Keyword recognition
Keyword extraction, or recognition, is an analysis technique that automatically extracts the most frequently used and important words and phrases from a text. It’s highly useful for automatically summarising content and better understanding the main topics discussed.
Sentiment analysis
Sentiment analysis aims at identifying the intentions and opinions behind the collected data. While text analysis makes sense the data set, sentiment analysis reveals the underlying emotions expressed through a statement or in a conversation. It reduces subjective feelings to abstract but processable representations. As with any data analysis, however, some nuances get of course lost along the way.
You want to test Tucan.ai for your Company?
More dynamic and authentic insights
Not only can with the help NLP additional and better qualitative insights be gained, interviews and analyses can also be made much more context-sensitive – for example, because interviewers and moderators follow a script that is continuously adapted by a computer.
NLP offers a multitude of new possibilities for optimising research processes and getting a deeper, more dynamic and authentic understanding of collected data. We will likely soon see much more conversational surveys where follow-up and in-depth questions are asked in real time.
That much is clear: AI is about to revolutionise qualitative research. It is not (yet) able to mimic the deep, explorative abilities of humans. However, we have undoubtedly reached a point at which some programmes can execute certain tasks much faster, cheaper and more reliably than humans.
Florian Peschl
florian.peschl@tucan.ai